According to McKinsey’s April 30, 2021 article: Succeeding in the AI supply-chain revolution, the successful implementation of Artificial Intelligence in Supply Chain management is estimated to have enabled early adopters to reduce logistics costs by 15%, minimize inventory levels by 35% and improve service levels by 65%.
The merits of Artificial Intelligence are constantly being promoted in supply chain. Yes, but in concrete terms, how does this artificial intelligence technology benefit our sector?
This article highlights the need to understand the practical application of Artificial Intelligence in the Supply Chain, revealing different faces of this revolutionary technology.
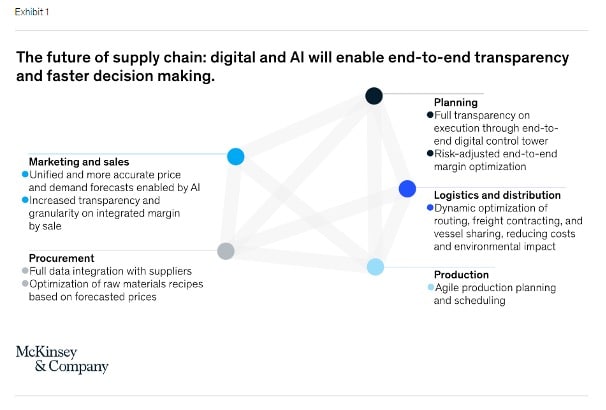
The foundations of Artificial Intelligence
One of the cornerstones of AI is the concept of machine learning. Machine learning algorithms enable systems to learn from data and improve their performance over time without being explicitly programmed. In this section, we’ll understand these mechanisms and see how they apply to the supply chain.
Machine Leraning (ML) and Deep Learning (DL)
Machine Learning (ML), also known as automatic learning, uses algorithms to detect patterns in data and draw predictive analyses from them. In other words, the machine can automatically learn a set of rules from data feeds, without the need for specific prior programming.
Deep Learning (DL) uses an artificial neural network made up of several layers of neurons, each of which interprets information from the previous layer to advance the system’s learning. Like Machine Learning, Deep Learning requires training with large datasets, and its accuracy increases with the size of the data used.
Optimizing transport operations
In delivery route planning, AI uses machine learning, IoT and GPS technologies to proactively manage transport in real time. It optimizes routes for maximum efficiency.
It provides a clear view of requirements, enabling more efficient allocation of resources and optimization of logistics routes.
Optimizing picking paths
AI is used to optimize slotting, determining the best product locations and picking paths to reduce order-picking time. Its predictive aspect ensures proactive preparation in anticipation of future demands.
By scheduling tasks according to product availability, AI improves operational efficiency in the warehouse. It ensures smooth operations management by adapting to fluctuations in product availability.
Solving complex problems
AI helps automate the resolution of complex problems, simplifying decision-making. Its ability to process complex data enables rapid, accurate analysis of difficult situations.
Calculating and adjusting leadtimes
Given the vast amount of data in today’s society, enabling artificial intelligence to learn and automate data analysis to predict delivery times simplifies and improves this often difficult and time-consuming task.
Due to unexpected delays in the supply chain, making assumptions about delivery dates could be a costly mistake.
AI, with the use of intelligent sensors and GPS data, makes it possible to track the location of products throughout the distribution process, preventing errors and improving the accuracy of delivery times.
Invoicing and cash-flow prediction
Companies can also automate invoicing with predictive artificial intelligence analysis. Future balances can be calculated for new invoices thanks to intelligent technology that recognizes trends in incoming payments and company spending.
Risk Management
Artificial Intelligence can also mitigate risks in the supply chain. Its transparency and predictive capacity influence decision-making on multiple scenarios linked to risks and opportunities, such as fluctuations in demand linked to events internal or external to the company. These scenarios can also be established between suppliers and customers.
Automate certain tasks
AI will also enable teams to refocus on less repetitive activities, processing large quantities of data without drowning in detail. However, this will require the support and evolution of teams in terms of organization, skills and training.
Safety and ethics when using AI
The mainstream adoption of AI raises questions about data security. It also highlights the crucial importance of ethics and transparency in the development and application of AI solutions.
So be careful not to give in to the siren calls of a technology without having clearly defined its usefulness and objectives.…
Case study 1: Walmart, company pioneer in the use of AI
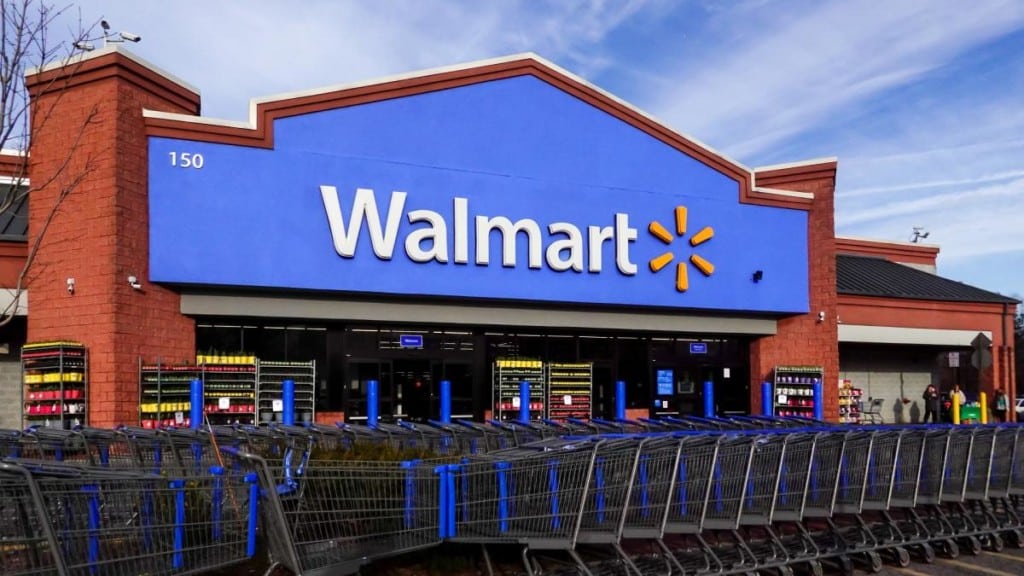
Walmart makes extensive use of AI in its supply chain, adopting a comprehensive approach covering automation, negotiations, purchasing and customer interaction.
By using AI in contract negotiations, Walmart’s chatbots (Pactum) have achieved purchasing cost savings of 1.5% and extended supplier payment terms.
Automation initiatives, targeting 65% store automation by 2026, include platforms such as Google’s BERT and GPT-4.
AI predictions improve supply chain workflows, balancing inventory during peak events such as Black Friday.
The technology helps reroute shipments during unforeseen events, such as Hurricane Ian, demonstrating Walmart’s agility.
According to the company, its investments in AI have resulted in substantial benefits, including a 15% reduction in logistics costs, a 35% minimization of inventory levels and a 65% improvement in their service levels compared to competitors.
Case study 2: Carrefour innovates with AI to optimize its supply chain and reduce waste
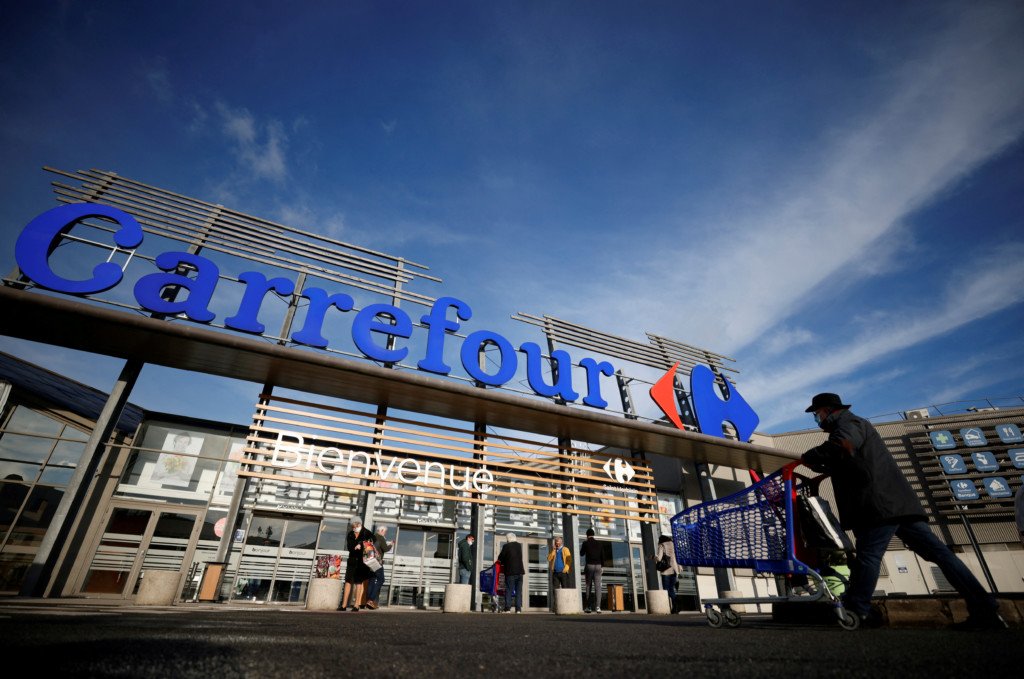
In 2023, Carrefour became the first French retailer to use artificial intelligence to optimize inventory management and reduce waste.
The AI solution collects and processes data from stores, warehouses and e-commerce sites to anticipate demand and fine-tune orders to suppliers. This smarter supply chain management ultimately leads to a reduction in out-of-stocks and overstocks in stores and warehouses.
The deployment of AI also enables the collaboration of several new professions involved in a complementary way in forecast management, such as the Data Scientist for data processing and the Demand Planner for business expertise.
Finally, it also enables Carrefour to go a step further in optimizing its Supply Chain, freeing up time for teams who can focus on developing differentiated forecasting strategies that best meet customer expectations while reducing waste.